AI and Predictive Sales Forecasting: A Future Guide
AI in predictive sales enhances accuracy, enabling real-time updates and optimizing processes for data-driven decisions.
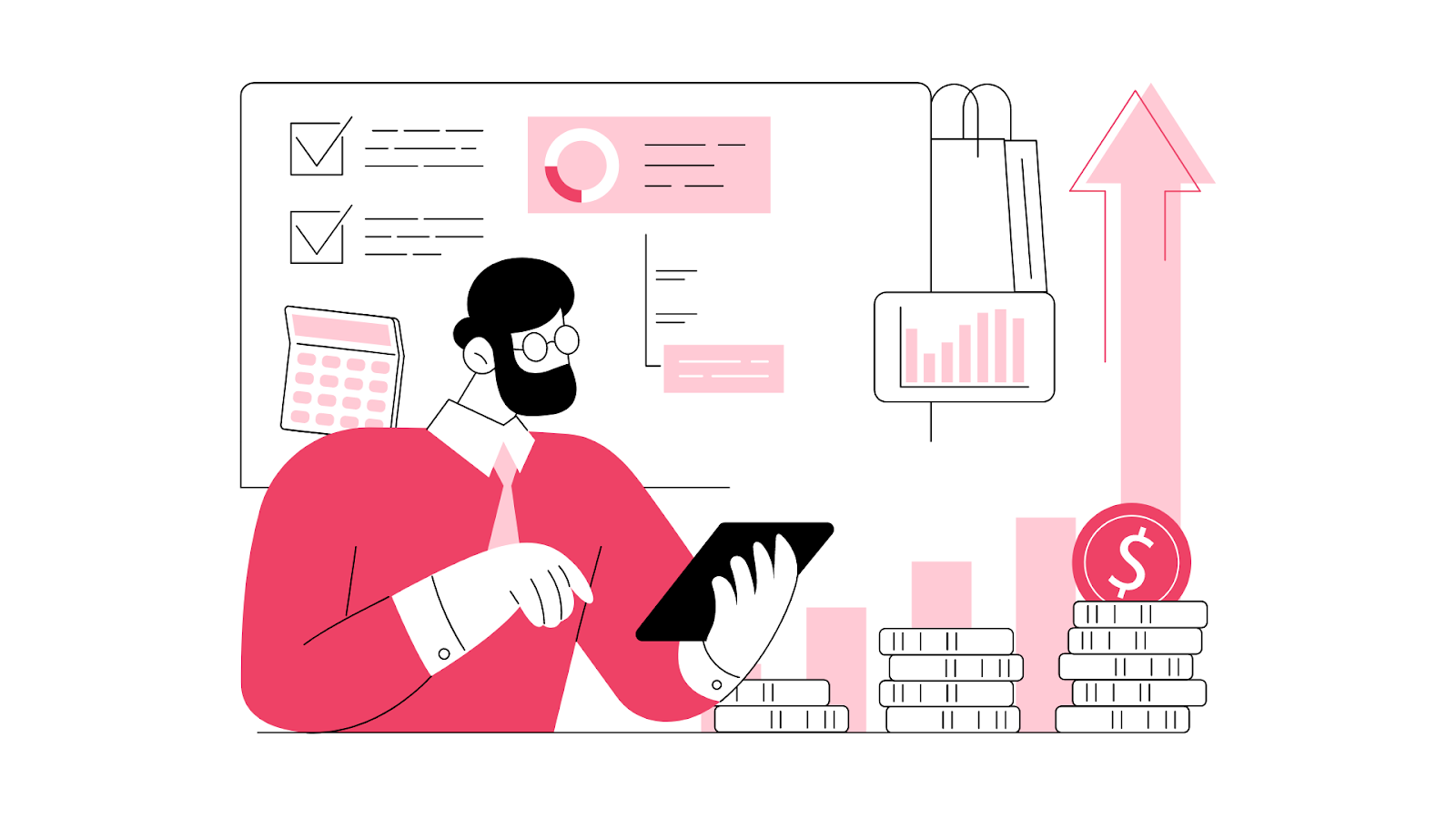
Sales forecasting is a fundamental part of company strategy that many companies need help with. Presently, most sales teams—especially those of small to medium-sized businesses (SMBs)—are less than 75% accurate in their sales forecasts, and 79% miss their sales forecast by more than 10%.
AI in predictive sales is emerging as a powerful tool transforming industries, from predictive marketing and self-driving cars to personalized recommender systems and intelligent virtual assistants. Among these innovations, AI in predictive sales forecasting has become crucial for businesses to improve accuracy. According to sources, firms using AI in predictive sales forecasting tools can achieve more than 75% accuracy, with specific studies showing an 82% increase in forecast precision.
What is Predictive Sales AI Forecasting?
AI in Predictive sales forecasting involves using historical data, market patterns, and external factors (like economic conditions) to forecast future sales. AI/ML Models can analyze data much faster and more accurately than humans, giving businesses a clearer picture of what to expect in the coming weeks, months, or years.
Role of Predictive AI in Sales Forecasting
AI in predictive sales tools provides various insights into CRM (Customer Relationship Management) and ERP (Enterprise Resource Planning). These integrations are incredibly useful in sales forecasting, utilizing AI in predictive sales to minimize the time required for complex data analysis.
CRM systems equipped with AI in predictive sales can help sales teams determine which leads are most likely to convert. On the other hand, ERP systems provide extensive insights into product demand and stock needs, permitting better planning and resource allocation. Meanwhile, adopting AI in predictive sales forecasting tools transforms sales within both ERP and CRM departments, driving profitability and efficiency.
End-to-End CRM to Slack Integration of Data: How to Achieve Seamless Data Sync: CRM to Slack Integration (floworks.ai)
Implementing Predictive AI in Sales Forecasting
Predictive sales forecasting utilizes various methodologies to enhance the accuracy and reliability of sales predictions. Here are some essential methods commonly employed:
Source: Link
Goal Setting for Specific Project
Businesses must define clear objectives before implementing predictive AI in sales forecasting. This is essentially the first stage of requirement analysis for AI in predictive sales forecasting, especially for small businesses. Are they looking to improve forecast accuracy, optimize resource allocation, or enhance customer retention?
Setting specific goals ensures that AI in predictive sales aligns with the company’s overall strategy and allows success to be measured effectively.
Data Preparation
Data is the foundation of any AI-driven system, and it is essential to collect, organize, and clean critical sales data before feeding it into the AI sales forecasting tools.
This might include historical sales data, customer interaction logs, and external data like market trends. This can be further divided into two sub-processes:
Step 1: Acquire and Organize Data
The first step in this process is to acquire and organize data. This involves collecting and storing relevant data from various sources from:
- Internal databases - Sales records for the past three years, including volume, product categories (e.g., t-shirts, jeans, jackets), and pricing.
- External APIs - Seasonal trends, marketing campaigns, economic conditions (like inflation rates), and even weather data (because yes, weather can influence shopping habits—especially for clothing).
- Web scraping - Data from the websites
- Surveys - Purchase history, demographics (age, location, gender), and website interaction data.
- Sensors - Sensing related data like temperature, wind, humidity, and AQI.
The organized data should be housed in a repository like a data warehouse or a data lake, which supports large-scale data processing and analysis.
Step 2: Pre-Process Data
Once the data is collected, the next step is to pre-process it. This includes cleaning and preparing the data for predictive modeling by
- Removing anomalies - If the same customer appears multiple times with slightly different names (e.g., "John Smith" and "J. Smith"), clean up these records to avoid skewing your data.
- Missing value - If a customer’s location is missing but you have it from a previous purchase, use that information to fill in the blanks.
- Outliers - Errors that could affect the quality of predictions.
Once the data is cleaned up, organize it in a way that makes sense for your AI sales forecasting. This might mean structuring sales data by month or quarter, or organizing customer data by demographics to make analysis easier.
Predictive Sales AI Model Training
Once the tools are selected, AI models must be trained using historical data. This training allows the AI to learn from past sales trends and customer behaviors, enabling it to make accurate future predictions. Think of it as teaching a new AI sales rep about your company's history to predict future sales.
Deployment and Monitoring: Keeping Your AI Sales Forecasting System Performing
Once your AI model is trained, it's time for deployment—integrating it into your sales forecasting system. Think of it like giving your AI model a driver's license—trained and ready to navigate real-world data, making predictions based on what it has learned.
But just like a car needs to fuel, your AI model requires continuous monitoring. Monitoring involves tracking prediction accuracy and real-time performance and making necessary adjustments, like retraining or tuning hyperparameters, to keep it on track.
Deployment Metrics
- Integration Efficiency: Measure how smoothly the model integrates with existing workflows.
- Latency: Track the time it takes to generate predictions; ensure it's suitable for real-time decisions.
- Scalability: Monitor if the model can handle increasing data without slowing down.
Monitoring Metrics
- Forecast Accuracy: Compare predictions against actual outcomes, aiming for at least 75% accuracy.
- Precision and Recall: Gauge how well the model identifies true positives and its sensitivity to relevant instances.
- F1 Score: Delivers a balance between precision and recall.
- MAE: Measures the average prediction error.
- RMSE: Highlights sensitivity to more significant errors.
Remember, markets change, and so does data. Ongoing monitoring ensures your AI evolves with the environment, maintaining its competitive edge.
Feedback Loop
AI doesn't just predict sales—it learns and improves over time through the feedback loop. Here's how it works: new sales data comes in after your AI model makes predictions.
That data is then fed back into the model, allowing it to fine-tune and adjust future predictions based on fresh insights. This continuous cycle makes the AI more intelligent, much like a kid learning to ride a bike—they get better with practice and correction.
The Process of a Feedback Loop:
- Initial Prediction: AI makes sales forecasts based on historical data.
- New Data: Actual sales results are collected.
- Comparison: Predictions are compared to actual outcomes.
- Adjustment: AI adjusts its model based on any discrepancies between predicted and actual sales.
- Refinement: The model improves and generates more accurate predictions next time around.
This loop continues, ensuring the AI adapts to changing trends and becomes more precise.
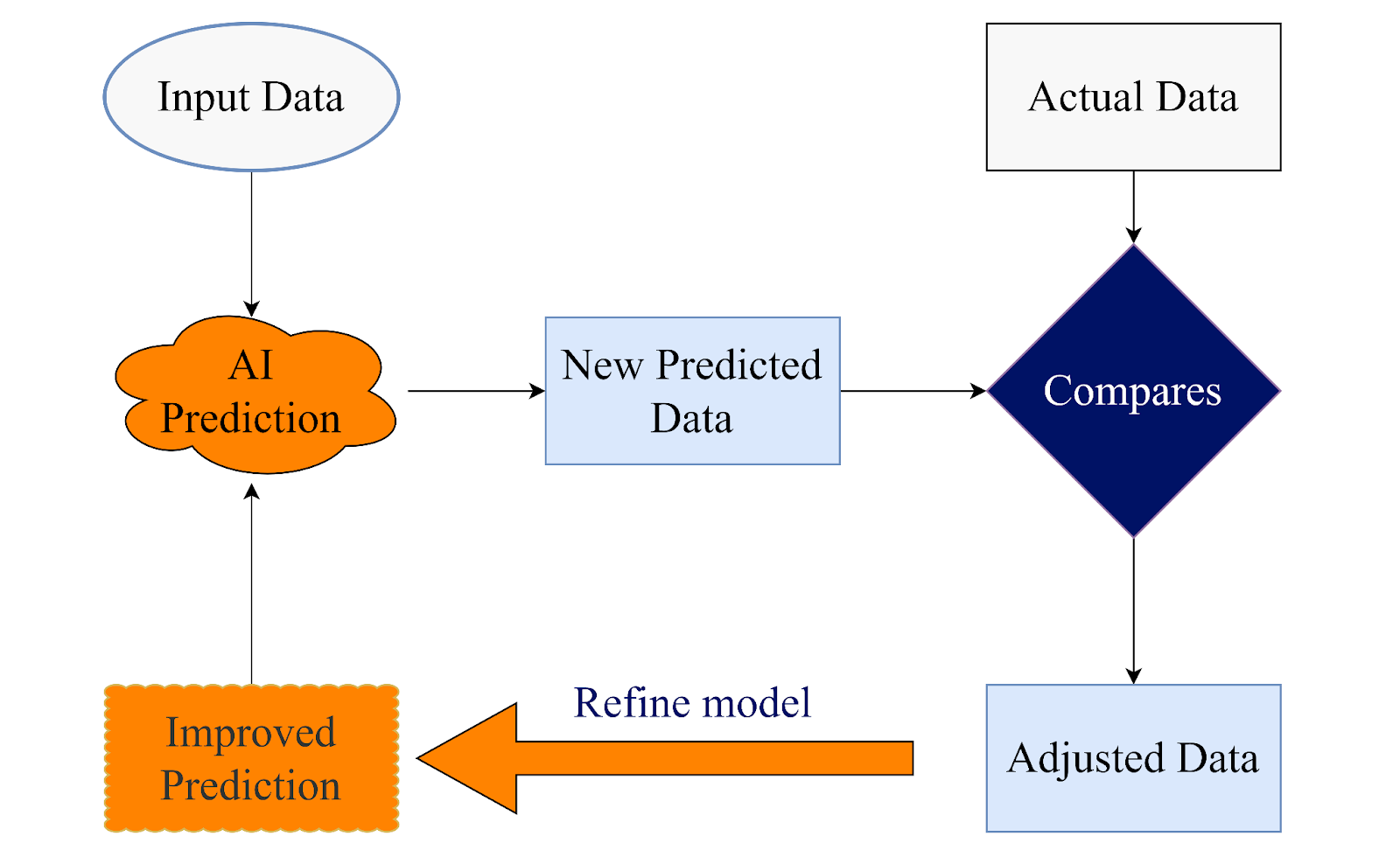
By continuously feeding your AI new information, you ensure its predictions grow sharper, keeping your business one step ahead!
Which AI sales forecasting tools should I use?
Choosing the right AI sales forecasting tools can revolutionize your strategy, helping small businesses optimize sales automation by integrating seamlessly with existing systems like CRM or ERP.
- AI is revolutionizing the sales landscape, mainly through predictive sales forecasting, which is essential for small businesses looking to enhance their AI sales automations and strategies.
- AI in predictive sales can be identified as per the project or the requirements of the organization that uses AI for sales forecasting.
- When considering AI tools for sales forecasting, it's important to choose solutions that integrate smoothly with your existing systems.
- Whether you're working with a CRM, ERP, or a custom solution, seamless integration is key.
- For instance, tools like Floworks AI SDR can serve as an alternative to human sales development representatives, streamlining operations and improving efficiency.
Key Benefits of Using AI in Sales Forecasting and Their Applications
AI in sales forecasting offers a range of benefits that go beyond traditional methods, from identifying high-potential leads to making real-time adjustments based on market shifts.
Lead Identification and Qualification:
- AI tools can predict the likelihood of leads converting into sales. This helps sales teams prioritize high-potential leads, ensuring their efforts are focused on opportunities most likely to close, ultimately boosting conversion rates.
Retention Rate Improvement:
- By monitoring customer activities and interactions, AI identifies customers at risk of churning. Businesses can then engage proactively with these customers, offering targeted solutions or incentives to enhance retention and reduce turnover.
Customer Sentiment Analysis:
- AI-driven tools analyze customer feedback, reviews, and behavior to gauge sentiment. Understanding customer emotions allows businesses to tailor their interactions, providing personalized experiences and improving customer satisfaction.
Enhanced Prediction Accuracy:
- AI minimizes human errors by analyzing large datasets and noticing patterns that might otherwise be ignored. This results in highly accurate sales forecasts, enabling better business planning and resource allocation.
Real-Time Adjustments:
- AI enables dynamic forecasting, allowing businesses to make real-time adjustments based on varying market conditions or customer behaviors. This flexibility ensures businesses remain agile in responding to external shifts.
Optimized Sales Processes:
- By automating regular tasks such as data entry, lead scoring, and reporting, AI frees up valuable time for sales teams. This allows them to focus more on strategic analysis and customer engagement, driving greater efficiency.
Data-Driven Decisions:
- AI provides reliable, actionable insights, empowering businesses to make strategic, data-backed decisions that are more informed and impactful.
Challenges and Considerations in Predictive Sales Forecasting
While the benefits of artificial intelligence in predictive sales are significant, there are challenges to consider:
Data Quality:
AI systems require high-quality data to produce accurate forecasts. Poor data can lead to misleading insights.
Integration Complexity:
Merging AI with existing systems can be complex and requires technical expertise.
Ethical Concerns:
The use of AI in sales forecasting raises questions about privacy and data security that must be addressed to maintain trust.
Awareness and proactive management of these challenges are essential for successful implementation.
Conclusion
AI in predictive sales forecasting is a game-changer for small businesses looking to enhance their strategies. AI in predictive sales provides accurate predictions, analyzes customer behavior, and identifies hidden trends. Using AI to forecast sales helps allocate resources more efficiently and improves customer retention. Additionally, sales forecasting with AI in predictive sales allows for real-time adjustments based on market changes, leading to optimized processes and data-backed strategies that drive growth and profitability.
For small businesses, AI-powered sales forecasting can automate routine tasks and provide real-time insights. Tools like Alisha AI SDR , simplify sales and business forecasting AI by managing leads and automating mundane tasks. With its seamless integration into your CRM, the sales team can focus on strategy rather than manual processes.
Using AI for sales forecasting ensures you're always ahead of the curve. Start transforming your sales strategy today with AI sales forecasting tools and ensure you stay competitive in an ever-changing market. Contact us now!
Frequently Asked Questions (FAQs)
How is AI used in sales forecasting?
AI analyzes historical data, identifies patterns, and forecasts future sales more accurately. This reduces reliance on guesswork, helping companies optimize inventory levels, streamline supply chains, and meet customer demand more efficiently.
What is the future of sales with AI?
AI will revolutionize sales by automating tasks like lead scoring, customer segmentation, and personalization. By 2025, AI-powered sales tools are expected to automate up to 40% of administrative tasks, allowing salespeople to focus more on making relationships and closing deals.
How can AI be used in forecasting?
AI improves forecasting by analyzing large datasets and learning from patterns over time. It can predict seasonal trends, customer behavior shifts, and external factors like market conditions, enabling businesses to plan more effectively for future demand.
Which method is used to predict future sales?
Standard AI-driven methods for sales prediction include time-series forecasting, regression models, and machine-learning algorithms. These techniques analyze past sales data and external variables to generate more accurate forecasts than traditional methods.
How does AI predict the stock market?
AI uses advanced algorithms to analyze real-time market data, financial reports, and news and social media sentiment. Some AI systems have been able to predict market trends with greater accuracy than traditional models, offering valuable insights for investors.
What are the benefits of AI demand forecasting?
AI demand forecasting enhances accuracy, reduces inventory waste, and helps prevent stockouts. According to a study, businesses using AI for forecasting see a 30-50% improvement in forecast accuracy, leading to positive customer satisfaction and cost savings.
Will AI replace salespeople?
AI will not fully replace salespeople but will support them by automating repetitive tasks such as data entry, lead scoring, and customer outreach. This will let sales teams to focus on high-value tasks like relationship building and negotiations.
How accurate are AI predictions?
AI predictions are generally more accurate than traditional models when they have access to large, high-quality datasets. Studies show that companies using AI for sales forecasting can reduce errors by up to 40%, making it a valuable tool for improving sales performance.
What is the AI market forecast for 2025?
The AI market is expected to reach over $190 billion by 2025, driven by increased healthcare, finance, and retail demand. AI in sales forecasting will play a significant role, helping companies improve efficiency and optimize strategies.
Why does AI in retail create better demand forecasting?
AI improves retail demand forecasting by analyzing consumer behavior, market trends, and external factors. This allows retailers to stock more accurately, reducing excess inventory and improving customer satisfaction by ensuring product availability.
Discussion